
​
​
Multi-Scale Embodied Intelligence Lab
Intelligent Robotics Across Scales
email: d.zhang17@imperial.ac.uk
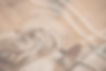
Intelligent Dexterous Manipulation
Here shows our work related to Intelligent Dexterous Manipulation.

Tac-VGNN: A Voronoi Graph Neural Network for Pose-Based Tactile Servoing
Wen Fan, Max Yang, Yifan Xing, Nathan F. Lepora, Dandan Zhang*
​
Paper Link: https://arxiv-export3.library.cornell.edu/abs/2303.02708
Accepted by 2023 ICRA
Tactile pose estimation and tactile servoing are fundamental capabilities of robot touch. Reliable and precise pose estimation can be provided by applying deep learning models to high-resolution optical tactile sensors. Given the recent successes of Graph Neural Network (GNN) and the effectiveness of Voronoi features, we developed a Tactile Voronoi Graph Neural Network (Tac-VGNN) to achieve reliable pose-based tactile servoing relying on a biomimetic optical tactile sensor (TacTip). The GNN is well suited to modeling the distribution relationship between shear motions of the tactile markers, while the Voronoi diagram supplements this with area-based tactile features related to contact depth. The experiment results showed that the Tac-VGNN model can help enhance data interpretability during graph generation and model training efficiency significantly than CNN-based methods. It also improved pose estimation accuracy along vertical depth by 28.57% over vanilla GNN without Voronoi features and achieved better performance on the real surface following tasks with smoother robot control trajectories.
For more project details, please view our website: https://sites.google.com/view/tac-vgnn/home

TIMS: A Tactile Internet-Based Micromanipulation System with Haptic Guidance for Surgical Training
Jialin Lin, Xiaoqing Guo, Wen Fan, Wei Li, Yuanyi Wang, Jiaming Liang, Weiru Liu, Lei Wei, Dandan Zhang
Full Paper Link: https://arxiv.org/abs/2303.03566

Microsurgery involves the dexterous manipulation of delicate tissue or fragile structures such as small blood vessels, nerves, etc., under a microscope. To address the limitation of imprecise manipulation of human hands, robotic systems have been developed to assist surgeons in performing complex microsurgical tasks with greater precision and safety. However, the steep learning curve for robot-assisted microsurgery (RAMS) and the shortage of well-trained surgeons pose significant challenges to the widespread adoption of RAMS. Therefore, the development of a versatile training system for RAMS is necessary, which can bring tangible benefits to both surgeons and patients.
In this paper, we present a Tactile Internet-Based Micromanipulation System (TIMS) based on a ROS-Django web-based architecture for microsurgical training. This system can provide tactile feedback to operators via a wearable tactile display (WTD), while real-time data is transmitted through the internet via a ROS-Django framework. In addition, TIMS integrates haptic guidance to `guide' the trainees to follow a desired trajectory provided by expert surgeons. Learning from demonstration based on Gaussian Process Regression (GPR) was used to generate the desired trajectory. User studies were also conducted to verify the effectiveness of our proposed TIMS, comparing users' performance with and without tactile feedback and/or haptic guidance.

Robot Learning for Service Robots
Learning from Demonstration for Automatic Tea Preparation for Service Robots
AARON ASAMOAH; Virginia Ruiz Garate; Dandan Zhang